Anonymized EMR-based Data Analysis – another look
In a recent post, we described how analyzing anonymized data that comes from Electronic Health Records (EHRs), and cross-referencing this data with other large public data sources, is the next “big thing” in healthcare.
In hopes of encouraging innovation and discovery that can help spur rapid advances in our understanding of disease and best treatments, we have partnered with Microsoft’s Azure MarketPlace by offering a set of de-identified data to help with such research. In conjunction with the Health 2.0 Conference in San Diego this March, we are sponsoring a developer’s challenge – “Analyze This” – in order to encourage coders and analysts to discover new patterns in the emerging “big data.”
We previously showed some data correlating the incidence of diabetes as a function of increasing obesity (as measured by body mass index, or BMI). This data validated the already-known correlation – people who are very heavy (BMI over 40) have over a four-fold increased risk of having diabetes than people who have normal BMIs (below 26). This is an example of a correlation within a given data store.
Cross-mapping data from one source (anonymized EHR data) with other outside data sources (such as Census-bureau data) can yield more interesting results. As a “teaser” example, we calculated mean BMI for each zip code in the U.S. (where we have at least 10 valid adult BMI measures in that zip code), and cross-plotted them against Median Household Income for that zip code (using Census data, publicly available). The question being asked is this: Is obesity a function of socioeconomic status?
The results are interesting. Without carrying out the vigorous statistical analysis that would be needed for an academic study, we can visually inspect the scatter-graphs and see some patterns. We can make some interesting conclusion from this data:
- Obesity is slightly predicted by Median Household Income, but not strongly so.
- This correlation is seen for females, but not for males.
What might explain this? One can speculate extensively. The point is that the data suggests an observational pattern and is food for thought, and further investigation.
Our hope is that showing what kinds of things can be done with anonymized, aggregated data, and cross-referencing them with other available data sources (in this case, Census data), investigators and analysts will be spurred to dig deeper. This is how the “new frontier” of clinical knowledge will emerge, and we are now at that threshold.
Robert Rowley, MD
Chief Medical Officer
Practice Fusion EMR
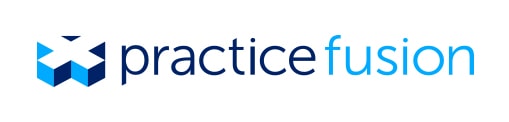